EMG-IMU Hand Gesture Recognition
Mobile devices such as smart phones, tablets and laptops are increasingly integral in our daily lives. Traditional interaction paradigms like on-screen keyboards can be an inconvenient and cumbersome way to interact with these devices. This has led to the advancement of bio-signal interfaces such as speech based and hand gesture based interfaces. Compared to speech input interfaces, hand gesture devices can be more intuitive in resembling physical manipulations related to spatial tasks such as navigation on a map or manipulating a picture.
Electromyography (EMG) is well suited for capturing static hand features involving relatively long and stable muscle activations. At the same time, inertial sensing can inherently capture dynamic features related to hand rotation and translation. This project explores a hand gesture recognition wristband based on combined EMG and IMU signals. Preliminary testing was performed on four healthy subjects to evaluate a classification algorithm for identifying four surface pressing gestures at two force levels and eight air gestures. Average classification accuracy across all subjects was 88% for surface gestures and 96% for air gestures. Classification accuracy was significantly improved when both EMG and inertial sensing was used in combination as compared to results based on either single sensing modality.
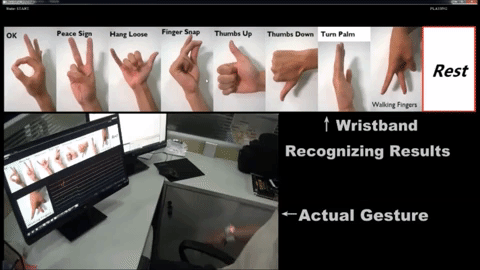
EMG-IMU Wristband for Hand Gesture Recognition
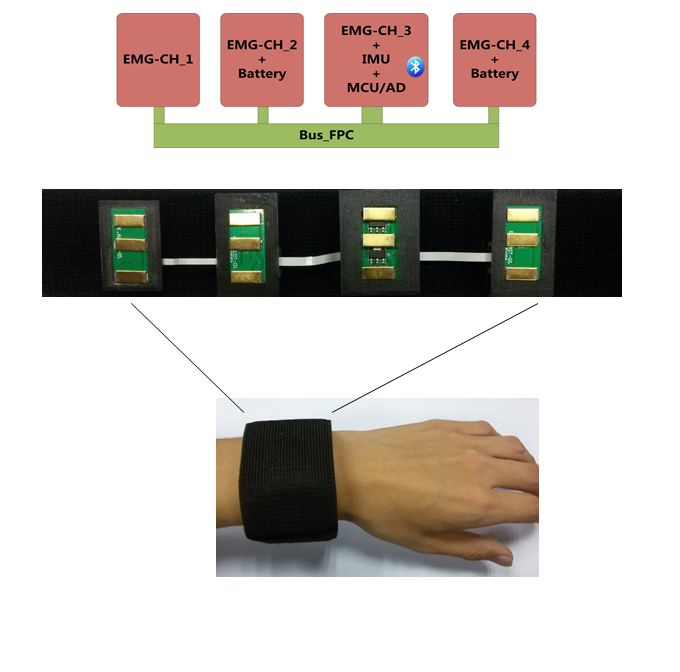
System Design
Overall structure of the hybrid EMG and IMU data acquisition wristband
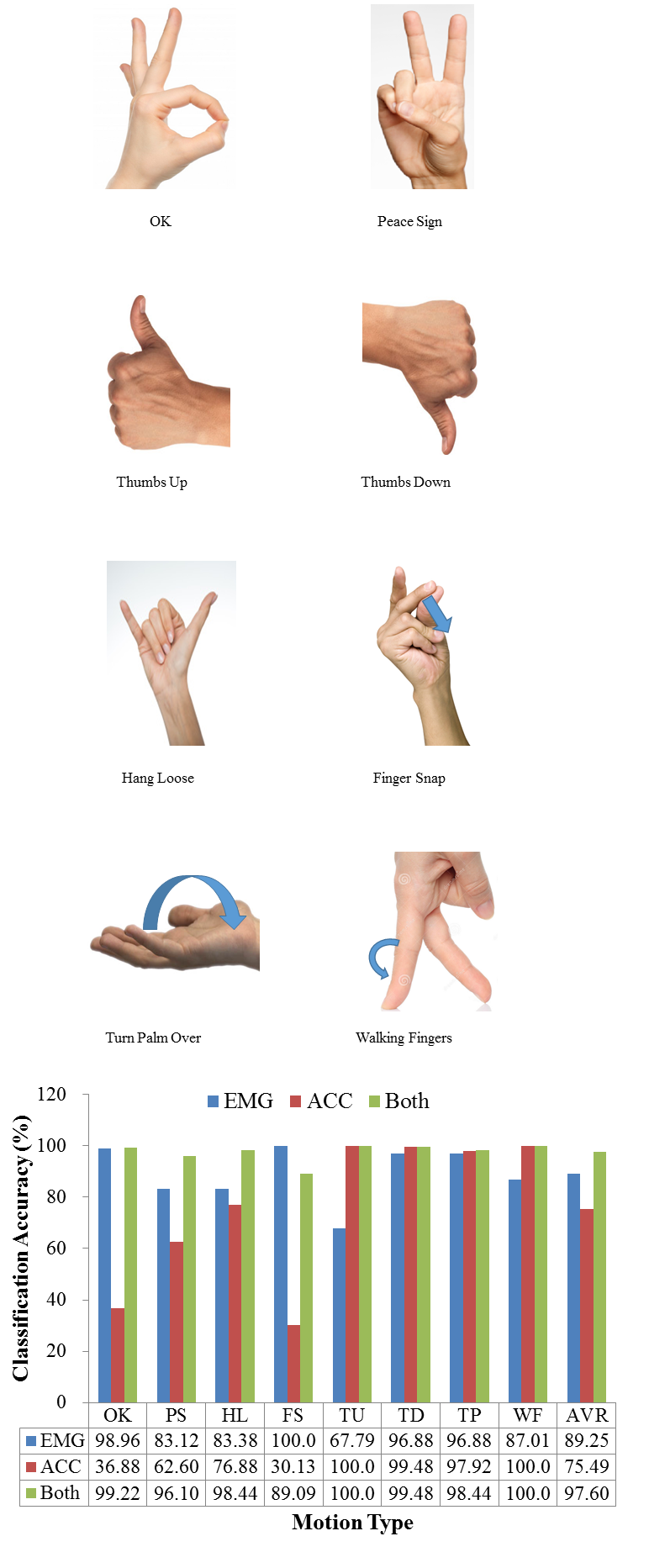
Air Gesture Recognition Rate
Classification accuracy of eight air gestures: okay sign (OK), peace sign (PS), hang loose (HL), finger snap (FS), thumbs up (TU), thumbs down (TD), turn palm over (TP), walking fingers (WF) for a representative subject. Classification accuracy is shown when using EMG or inertial sensing (ACC) individually, and in combination (Both).