Embodied Sensing
The focus of this research is to investigate the reconstruction of tactile perception information on the human palm during interaction through multimodal sensing on the wrist, a new wearable gesture recognition framework and object interaction posture sensing framework that does not rely on vision, and a novel soft somatosensory wristband based on a multimodal sensor array. Key scientific issues include solving the missing somatosensation for embodied perception problem, the inability to perceive hand gestures with tools interacting with the environment, and wearable hand and wrist perception limitations.
For robots that rely on visual perception, it is difficult to capture forces and torques at the end of tools. Humans however rely on somatosensation input and can perceive tool end forces and torques very well with little effort. This leads to a key important unsolved question: how to transfer the somatosensory perception capabilities from humans to robots?
Humans can comprehensively describe the kinematics, statics, spatial information, and intrinsic attributes of interaction processes solely using hand somatosensory perception. Robots currently can not do this. Thus, in this project we propose to perform 3 key endeavors. One: Invent novel electronics with skin-like perception properties. Two: Develop an interaction perception model based on somatosensation. And three: explore and implement novel imitation learning for manipulation strategy transfer.
This research involves developing novel electronics with skin-like perception properties that have high density, high driving ability, high operation speed electronics, and a low hysteresis, multi-dimensional, high tensile sensing mechanism. Intrinsically stretchable electronics will be followed by large scale flexible circuit and the development of ultra-low hysteresis soft sensing leading to complete e-skin for proprioception and tactile perception reconstruction.
An interaction perception model will be developed based on proprioception and tactile somatosensation perception. This involves compiling a multi-modal, large-scale human manipulation skills dataset and the development of efficient and physically accurate decoding strategy for somatosensory perception. Development of a human knowledge embedded dataset will be followed by decoding architectures for perception and the development of a semi-structured generative AI network leading to a perception model based on proprioception and tactile perception.
Finally, imitation learning for manipulation strategy transfer will be explored and implemented. This involves using a somatosensation guided manipulation generation strategy and a diffusion strategy for motion planning. Somatosensation guided manipulation generation will be followed by diffusion strategy motion planning and development scenario-based motion controller leading to dexterous manipulation with proprioception and tactile perception input.



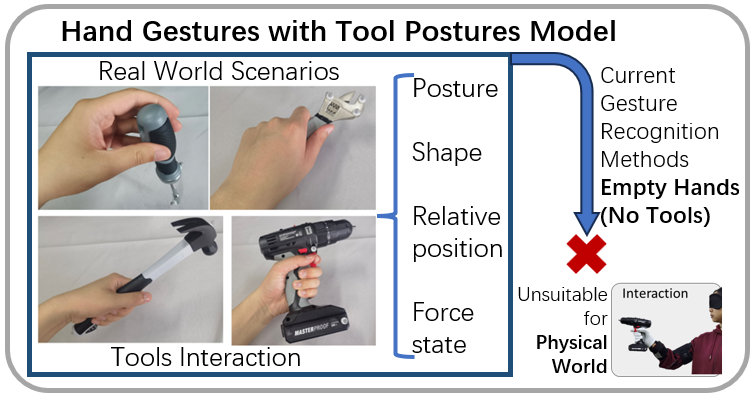
